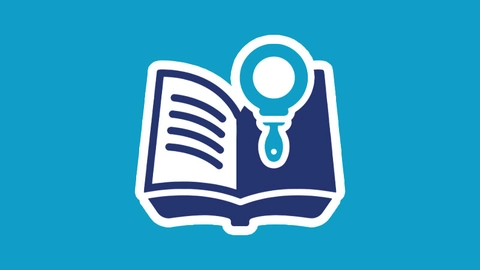
This publication highlights the development of an offline approach to discriminate different types of liver pathologies in rats called the “support vector machine”.
Key Points/Summary:
- Computer-aided diagnostic systems have been introduced to characterize normal vs. disease states in the liver
- Proposed a support vector machine (SVM) method
- Requires analysis of five independent features of the ultrasound scattering signatures that can result in more accurate disease classifications (fibrosis vs. inflammation)
- Their SVM produced a 94.6% classification accuracy
- RF (radiofrequency) data was collected
- Various signal processing techniques were used to analyze the liver echos including:
- H-scan classification
- Attenuation estimation
- B-scan intensity
- Ultrasound speckle statistics using the Burr distribution
- Various signal processing techniques were used to analyze the liver echos including:
- Vevo 2100 with MS250 transducer (21 MHz) was used for data collection
Baek J, Poul SS, Swanson TA, Tuthill T, Parker KJ. Scattering signatures of normal versus abnormal livers with support vector machine classification. Ultrasound in Med. & Biol., 2020; 00, 00. https://doi.org/10.1016/j.ultrasmedbio.2020.08.009.